Estimating Human PerceptionEstimating human perception using information from cameras and wearable sensors Estimating Human Perception
When explaining something to someone, we determine what we are going to say based on their level of understanding. Supposing an intelligent system could estimate a person’s level of understanding in this way, imagine what kinds of processes would make this possible.
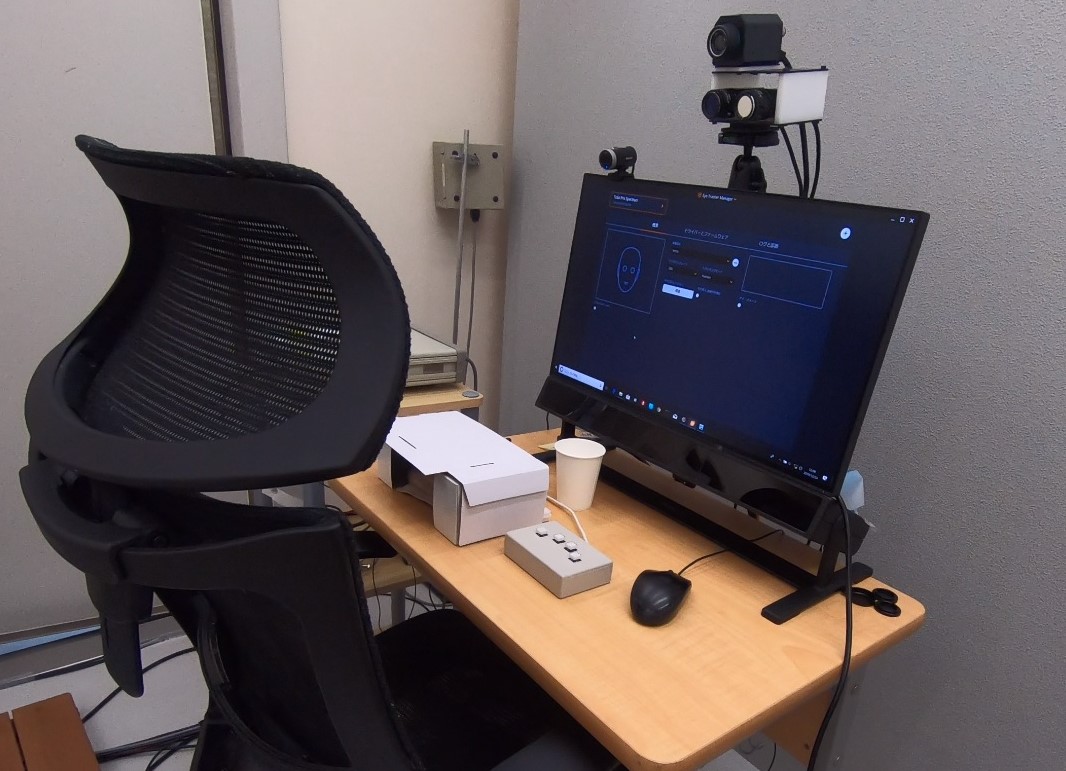
Index
Share
The ability to perceive whether an audience is understanding you is very important in communication
The purpose of this project is to estimate human perception. When AI is explaining something, does the audience understand what is being said? If not, maybe it would be better to explain it more simply. This happens naturally when humans communicate with each other.
Neuroscientific approaches, such as electroencephalograms (EEG) and fMRI, are common ways of examining human cognitive states like perception. However, since such approaches require fairly major equipment, they are not very practical for applying to robots and mobility in real life.
The focus of our research group has been on more practical techniques using cameras and wearable sensors. To begin with, cameras allow us to see facial expressions. Furthermore, it has been revealed that heart rate can be estimated by using image processing (rPPG) to detect slight color variations. And with thermal cameras, we can even see temperature distribution on a subject’s face.
Wearable sensors, on the other hand, allow us to acquire a variety of physiological data, such as blood flow, heart rate, skin potential and perspiration. In the future, it should also be possible to turn car steering wheels into wearable sensors.
Machine judges correctly in experiment as high as 80% of the time
Our research group conducted an experiment in which dozens of test subjects were asked to solve a problem. The problem was shown on a screen, and subjects pressed a button when they understood the solution. We looked for any changes before and after the moment they understood the solution, that is, the moment they pressed the button.
The results of analysis showed that heart rate tends to increase when someone solves a problem. This was modeled using machine learning, with the machine judging correctly whether a subject had solved the problem or not as high as 80% of the time based on heart rate data.
In order to check how machines compare to humans in making correct judgments, we had people look at the facial expressions of the test subjects and make the same “solved/not solved” judgment. On average, they judged correctly about 50% of the time. Although this was a simple experiment, it showed the potential for machines to achieve greater accuracy than humans.
We also analyzed facial expressions in this experiment, but no clear changes were observed before and after the button was pressed. This is probably because it is difficult for humans to express their emotions when they are dealing with machines. It may also be possible to detect the level of perception based on facial expressions when humans are communicating with each other.
Applications anticipated in various sectors, including business and education
The technology in the system developed by our research group for estimating perception could be applied to a wide range of purposes in the future. For example, when giving a presentation at work, knowing whether the audience is understanding or not is important information. By monitoring the level of perception and changing your wording accordingly, the probability of success can be increased.
At schools, COVID-19 has meant that classes are now being held remotely. One difficulty with this is that it is hard to tell from the screen whether students are understanding the lesson content. Using this system to estimate perception could help improve lessons.
In robots and mobility, this technology might also be useful for UI development. If the level of human perception for a presentation made by a machine can be quantified, it should be easier to improve the UI to make it more understandable.
Whereas the quiz experiment was an instantaneous stimulus, these application settings are basically continuous responses. Furthermore, communication between humans has the additional complexity that responses vary depending on their relationship and other factors. There are still many issues to be resolved in estimating perception, and a number of new innovations will be needed.
Nevertheless, in communication, ascertaining whether the other person has understood you or not is essential for making the relationship interactive rather than one-sided. If we can do this, AI should become an even closer presence to humans.
Voice
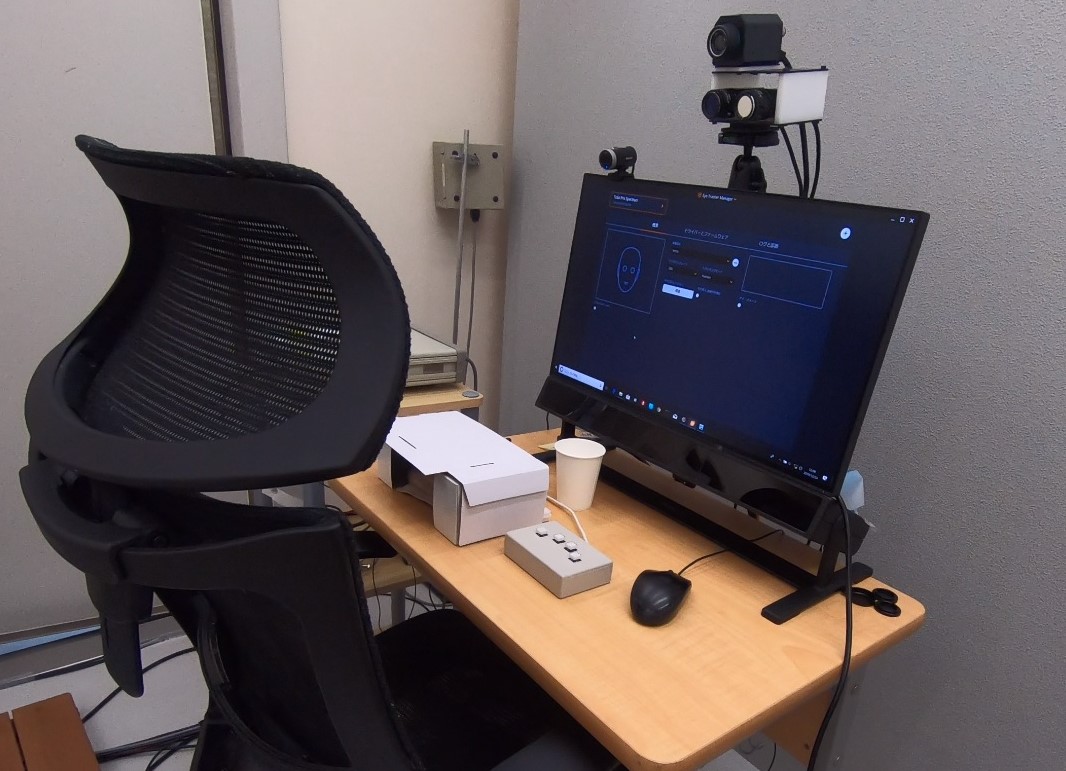
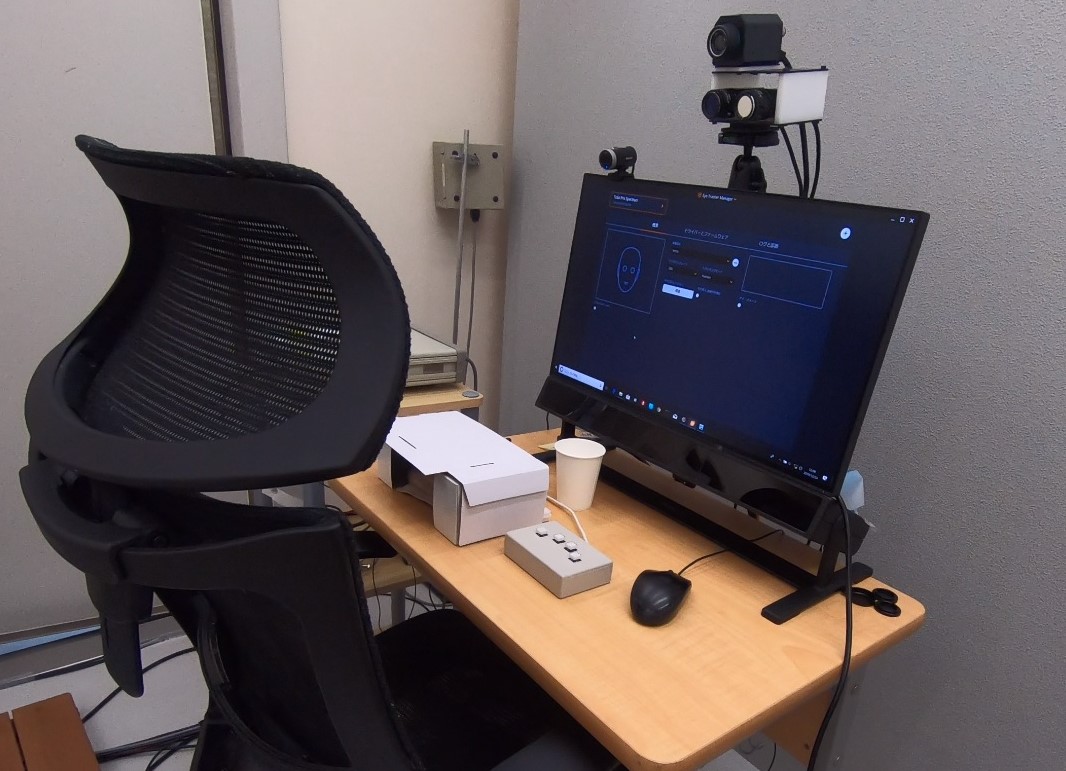
Perhaps because of Honda’s culture, I have connections not only with colleagues in HRI but also with specialists from various backgrounds across HRI-EU, HRI-US, Honda R&D and other places. They help broaden my own research area, networks and perspectives. I am drawn to this environment where I can work with a variety of people, not only in basic research, but also in needs-based research from an application aspect and in empirical research.